How to Embrace Opportunities with an Augmented Data Quality Strategy in 2022
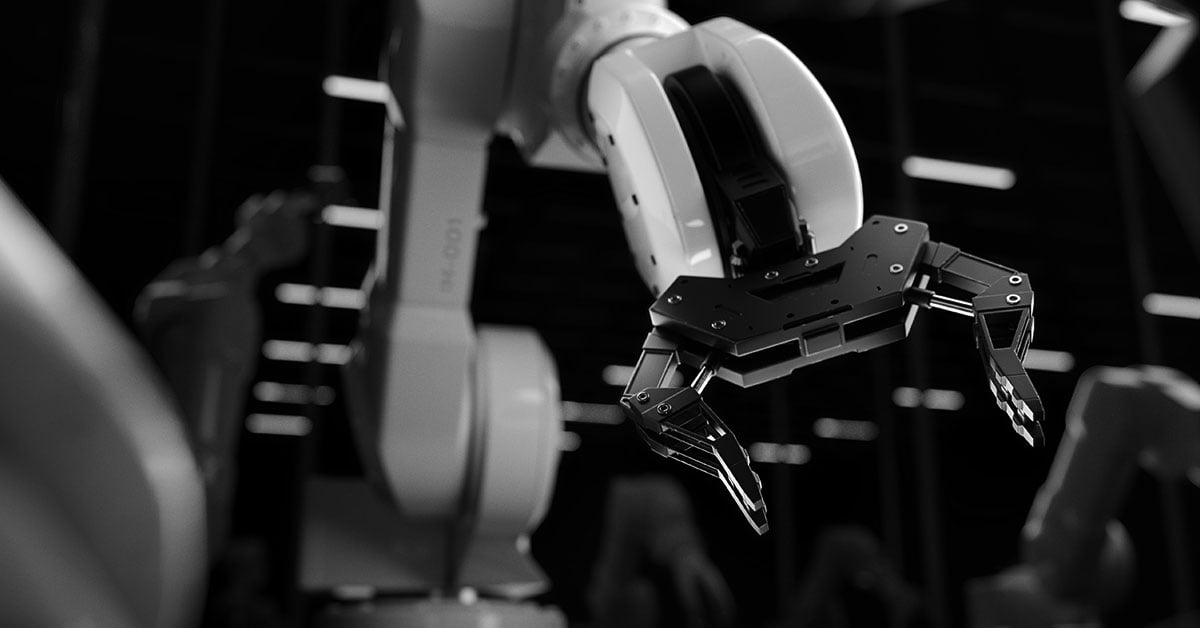
Gartner estimates that those organizations that rigorously track data quality levels via metrics will generate 60% more benefits across the board than their counterparts by the end of 2022.
Augmented data quality is a vital part of this rigorous process. There are four main reasons why manual data quality processes can no longer keep pace with the needs of modern organizations, and why an augmented data quality platform is essential.
- Degradation in data quality due to digital era expansion (anyone, any device, any location, any data, leading to too much noise)
- The classic “Garbage in leads to garbage out” issue, leading to bad predictions, poor outcomes and loss of investment
- A lack of consensus on the definition of data quality, with no systematic way to sustain high quality
- Operational cost of aiming to check and maintain even 50% data quality is quite simply too high - time and resources and tools and challenged with integration, metadata, curation, governance and master data management issues.
“Augmented data quality enabled by artificial intelligence offers organizations enhanced capabilities to automate process workflows and shorten time to value. Data and analytics leaders should use these capabilities and associated practices to optimize the value of data at enterprise scale.”
Source: Gartner
The Benefits of Augmented Data Quality
Augmented data quality reduces manual DQ processes and delivers enhanced data quality capabilities faster and at lower cost. This means that by investing in a clear, AI-driven data management strategy that includes the critical data capabilities needed to maintain high levels of data governance, data quality and metadata management, businesses can stay competitive and keep on a healthy growth trajectory in 2022 and beyond.
The continued increase in the volume of data being generated and consumed has meant that data management is now much more problematic and time-consuming than ever. Augmented data quality management is now a more sustainable option, rapidly gaining traction in the data science industry. Augmented data quality processes automate some of the data quality processes using active metadata and technologies such as artificial Intelligence and machine learning to shorten time-to-value and enhance the corporate decision making processes.
“By 2022, 60% of organizations will leverage AI-enabled data quality technology for suggestions to reduce manual tasks for data quality improvement”
Source: Gartner
What is Augmented Data Management?
Augmented data management utilizes ML technologies to enhance or automate core data management tasks, particularly time-consuming and data-intensive tasks which might normally be done manually.
The result is faster time to value with fewer errors, substantial cost reductions and improved decision making. Typical examples include spotting anomalies in large datasets, resolving data quality issues and tracing specific data from a report back to its origin.
Augmented Data Management in Practice
Augmented data quality allows you to automate many routine DQ tasks such as profiling, data matching, automatic linking between entities, merging, cleansing, monitoring, automatic alignment between business and IT control rules, troubleshooting anomalies or poor quality warnings. Its purpose is to cut down on manual tasks, reducing, but not removing, the need for human intervention in favor of automated workflows within processes and, consequently, saving valuable time and resources.
The principle is this: that skilled data quality professionals are freed up from manual tasks, and instead focus on value-added activities, confident in the knowledge and understanding that basic data quality rules have been automated, and can be applied at scale.
This can drive significant competitive advantage, as it gives management better line-of-sight to trends underlying the data.
Upscaling Data Quality Capabilities
Augmented data management accelerates many of the labor intensive tasks needed to deliver data certainty in three key areas of DQ capability: Discovery, Suggestion and Automation.
Source: Gartner ID G00719340 2020
*NLP: Natural Language Processing
AI will never eliminate the need for organizing the management of data within a company. Despite the many useful applications of AI, data management remains a people business, and the activities within will need a strong focus on change management efforts, cultural challenges and skills.
Augmenting part of the work can make that journey easier, but you will still need a dedicated team spearheading the data journey.
Implementing Augmented Data Quality Management
IDS’s latest data quality toolkit, iData 2.0, will be available for beta testing from mid-February 2022.
This, the latest version of IDS’s iData toolkit supports the fundamental requirements of augmented data quality management incorporating automation, machine learning and business intelligence to resolve critical data quality elements with minimal human intervention.
This latest release has a new and intuitive interface, making it easier for teams to collaborate on data quality initiatives, regardless of the size of the organization, or the complexity of the data lake.
To get your hands on iData 2.0 before anyone else, sign up to be part of the beta test program in February 2022.
Register your interest here today.

Learn how Continuous Testing can Improve your Business
IDS' Chief Technical Officer, James Briers, sheds light on the solutions to approaching complex data testing projects with mechanical efficiency.
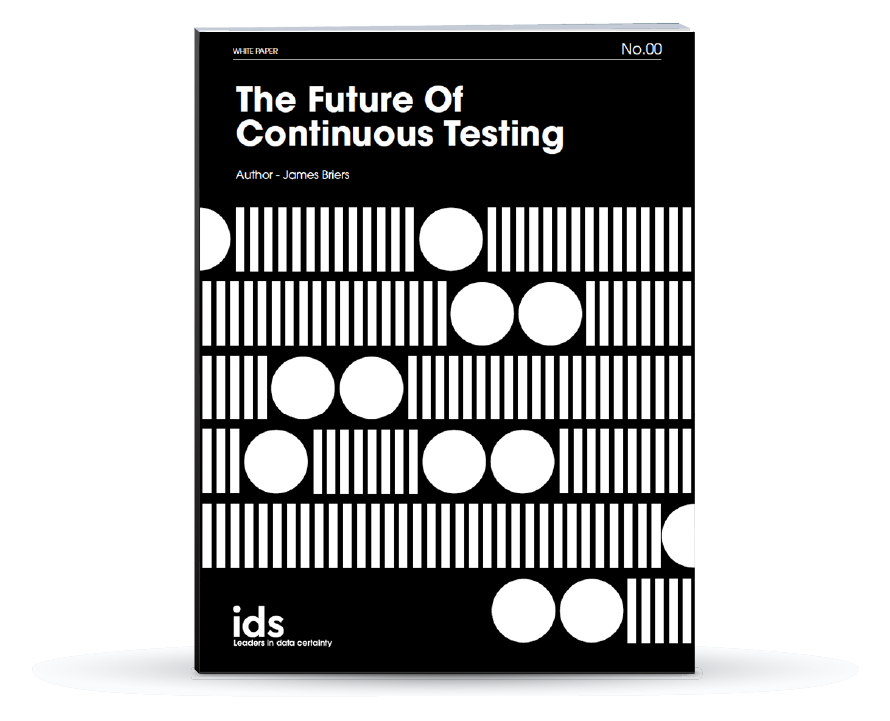