Financial Services
Data is the lifeblood of the insurance and finance industries.
From telematics data to credit checks, data quality in financial services is used to inform almost every decision.
It’s crucial that you have access to accurate and up-to-date data analysis to help you stay ahead of competitors and assess your portfolio of risk. In some cases, a lot of the data management in financial services can be littered with inaccuracies, as well as a lack of quality and testing. Data quality in insurance and financial services should be handled with care.
All too often, companies will mould together legacy systems with new architecture which can damage your ability to trace and process data effectively.
Improving Data Quality in Financial Services
Many companies in the insurance and financial sectors are under pressure to address data quality issues, but don't necessarily have the ability, time or resource to solve them quickly. The move to online platforms, as well as being at the mercy of comparison sites, has increased the amount of data available.
Data analysis and storage should be at the very heart of companies in this industry, in order to improve compliance, risk assessment and staff efficiency. In most cases, insurance and financial companies find themselves experiencing the following issues.
1. Lack of visibility around data quality
2. Lack of accountability and poor performance across campaigns
3. Difficulty recruiting the right talent
4. Inadequate or outdated testing services.
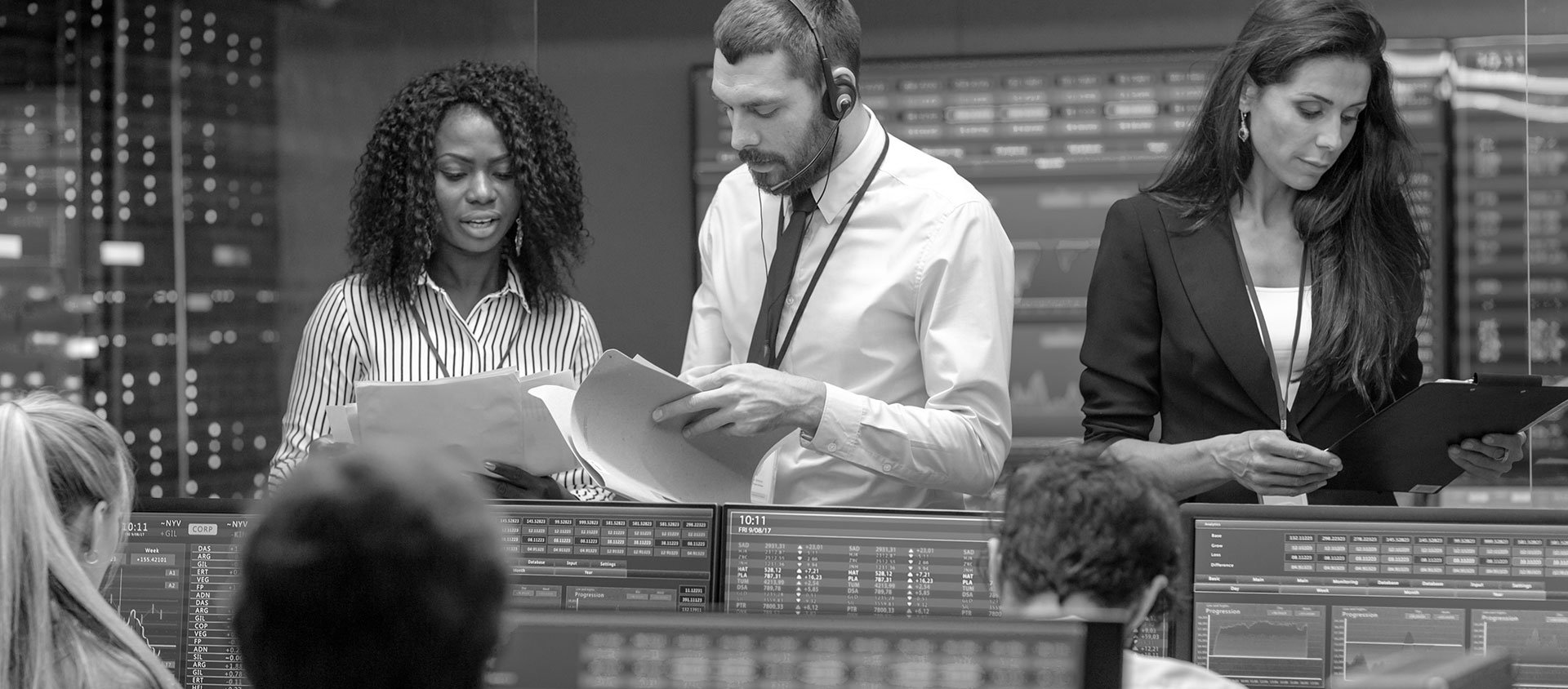
Data Certainty and the Customer Experience
Data trust, driven by a level of data certainty, is one of the key trends that will shape the banking sector in coming years. For banks and other financial institutions, the impact of big data on business centers around new levels of security and better customer loyalty driven by personalized offers and customer support.
Better customer service empowered by big data analytics is on the list of top priorities for the Royal Bank of Scotland (RBS). The RBS analyzes packaged bank accounts to detect duplicate payments and uses analytics to spot customers who could save money from consolidating debt. Their predictive analytics allow systems to send renewal reminders to mortgage holders when they approach an automatic transfer to a more expensive rate.
Data is Critical in the Banking Sector
Traditional banks, insurers and financial institutions have been shaken globally by the meteoric rise of FinTech brands that are quite literally stealing market share from beneath their feet. To compete, to grow, it is not enough to have financial weight, a large customer base, and investment expertise. How financial organizations use tech, and when and how they jump into new technology in a significant way, will determine their future success.
"The increasing role of user data to drive up the customer experience and net promoter score, as well as providing a single point of truth for strategic decision-making, is one of the most important areas.
Source: American Banker
- Turning visitors into leads.
Applications of iData in the Financial Sector
Applications of iData, and the Kovenant™ framework include:
- Big Data
- Data Governance
- GDPR Compliance
- Cost Reduction & Revenue Assurance
- Data Management
- Invoice Validation
- Dispute Management
- Revenue Assurance
- Interim Management
- Continuous Process Improvement
- Migration Projects
- ERP
- Data Visualization
- End-to-end data assurance.
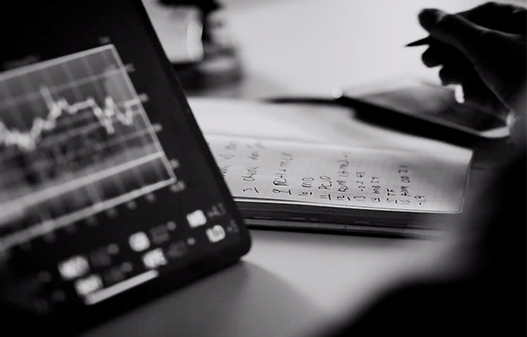
Risk Management Through Data Quality
Deeper data insights, driven by data trust, can help fraud prevention within the banking sector.
American Express, for example, reports a 100% improvement in its resolution rate for digital fraud and a 21% reduction in POS disruption since adopting AI-powered data analytics in 2014.
Risk management is a fundamental challenge for any financial organization. Singapore-based United Overseas Bank, for example, has reduced the time cycle of calculating the value at risk (the risk of loss for investments) from hours to minutes and Morgan Stanley has driven accuracy in financial risk prediction with the help of automated pattern recognition. Removing manual processes from data quality, migration and transformation, as well as testing and QA, is a critical step for any financial institution, to reduce risk.
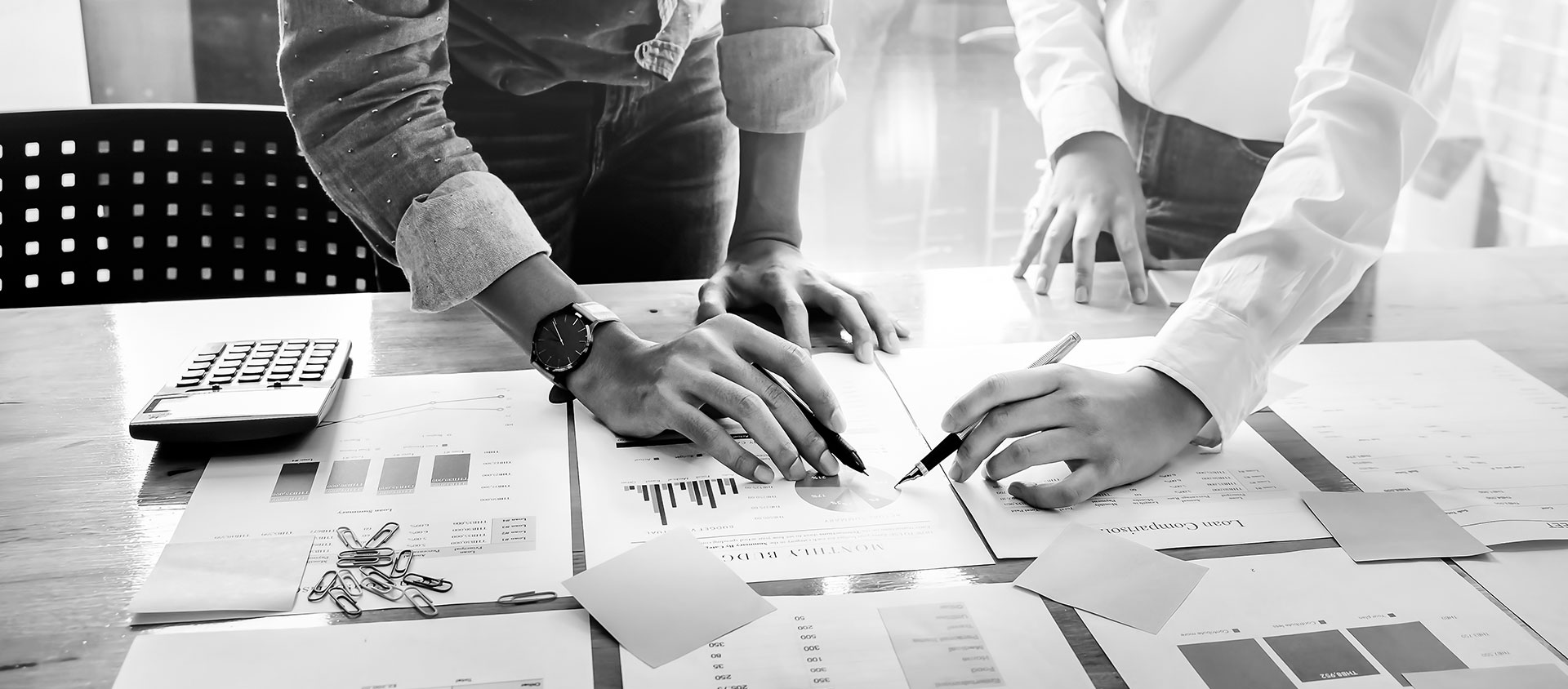
Data Governance and GDPR
Although banks prioritize data programs, their approaches to building data-management capabilities vary greatly in cost, risk, and value delivered. Increasingly, manual processes are being replaced by automated approached and supported by machine learning or augmented intelligence, such as IDS's testing automation solutions. However, in the absence of coordinated guidance from regulators, the banking industry takes a disparate approach to creating data-control frameworks, based on the risks that need to be managed and the pace of automation within a particular organization. This creates challenges around specific issues such as data governance and - for those operating in Europe - compliance with the GDPR (General Data Protection Regulation).