What is the Essential Element in the Data Analytics Process?
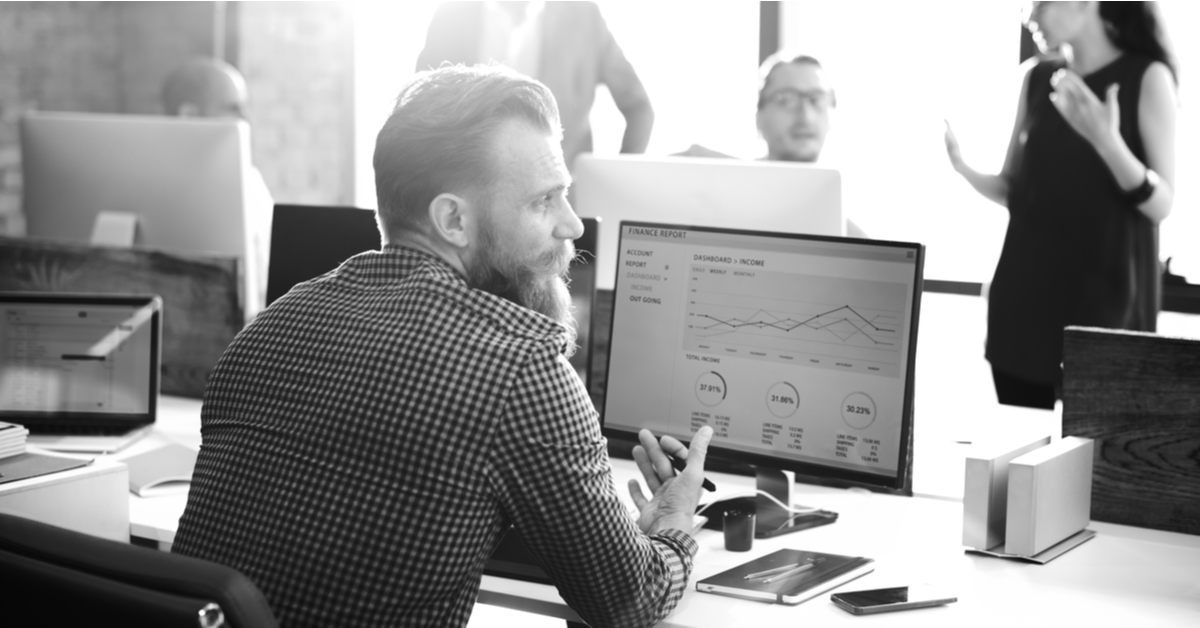
Enterprises of all types and sizes are increasingly reliant on the latest AI and ML based data analytics tools to support their strategic and operational decision making.
Whether that is to plan staffing levels, production schedules or manage supply chain partnerships. This makes the quality of the data the essential determining factor in the ultimate success of any business.
According to a Gartner report published in 2021 “Data quality is a key enabler for digital business initiatives” and recommends that
“Data and analytics leaders must collaborate with business stakeholders to establish effective and scalable data quality programs aimed at driving desired and sustainable business outcomes.”
This is clearly sound business advice and companies that fail to implement effective data quality programs are risking putting themselves at a serious disadvantage against their more data savvy competition. But it also raises the questions, what is data quality? what aspects of data should a data quality program focus on? and how can businesses assess the effectiveness of the investment and resources needed to implement it?
The Five Characteristics of Data Quality
When assessing the quality of data and whether it is fit for purpose business leaders need to look at five main characteristics:
Accuracy – Essentially, does the data look to be correct and conform to expectations that fit with normal day to day experiences?
Completeness – Does every record contain all the necessary information to make it useable? Are crucial parts of addresses or customer names missing?
Reliability – Are there any anomalies or differences between the same records in different data sets such as name spellings or birth dates?
Relevance – Do you still really need to retain all the data or has some of it outlived its original purpose or usefulness?
Timeliness – Is the data up to date? When was it collected and when was it last updated?
Issues with any one of these characteristics can have a very serious impact on the effectiveness of the analytic processes that, at best, can result in failure to generate meaningful insights and, at worst, lead to poor and ineffective management decision making.
Businesses have a range of options and best practices that can be deployed to ensure a data quality culture is engendered and enforced across the organisation and ensure that the worst case scenario does not become the norm. Data quality has the potential to impact virtually every employee’s ability to perform their role efficiently and effectively. It is therefore in the interests of everyone in the organisation that all data quality practices are not only followed religiously but the quality itself is routinely monitored and assessed on an ongoing basis. Without a rigorous approach to data quality it is virtually impossible to know if the investment in the DQM strategy is delivering an acceptable return.
A good indication of the success of the DQM strategy can be found by measuring the success rate of the data analytics processes. This can be measured both in terms of technical errors during analytics operations, but also anecdotally through the wider business KPIs, which if generally consistently trending upwards is a good indication that data underpinning the analytic processes is being maintained at the highest standards.
Another good and more readily quantifiable way of measuring effectiveness is in the data time-to value ratio. If data quality is poor this is likely to lead to slow data processing times with the inability to sustain a high volume data throughout as well as problems when it comes to transferring data from different formats. If data is inconsistent, the rate of data transformation errors are likely to be unacceptably high.
Implementing a Scalable and Effective DQM Strategy
IDS has developed a unique iData system and Kovenant™ methodology that has been deployed to provide the DQM and analytics platform for many sector leading enterprises across a broad range of different business sectors.
Providing business leaders with 100% data quality certainty 100% of the time, IDS helps to deliver trust in their analytics processes and confidence in their ability to make the right decisions that work for their businesses.

Learn how Continuous Testing can Improve your Business
IDS' Chief Technical Officer, James Briers, sheds light on the solutions to approaching complex data testing projects with mechanical efficiency.
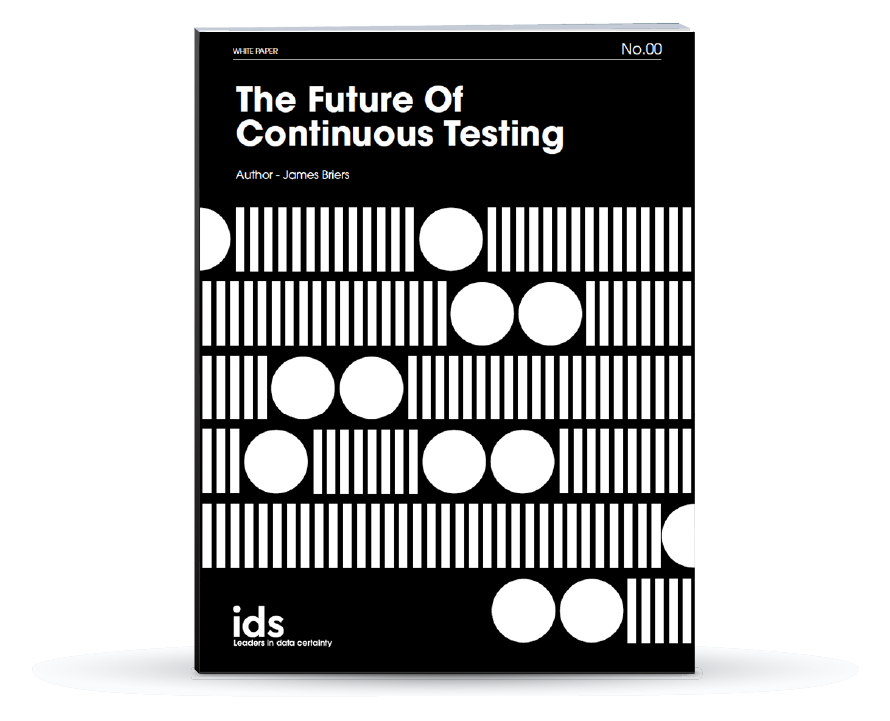