Data Quality Management
Data Quality Management Has Existed for a Long Time
In the year 1865, Professor Richard Millar Devens established the term business intelligence in his Cyclopædia of Commercial and Business Anecdotes. He used the term to describe how Sir Henry Furnese gathered information, and then acted on it before his competition did, to increase profits.
As we stand here in 2022, the reasons why data quality management are important remain exactly the same – to generate the information and insights needed to support decision-making. To give a single point of truth based on data certainty.
However, the world has changed. Data is bigger, more complex, and constantly changing. Technological change, consumer demand, environmental concerns and – of course - the global pandemic, have required organizations, industries, governments and conglomerates to aggregate, augment and respond to information, fast. This is widely known as the fourth industrial revolution.
The response to this level of change needs to be based on the highest levels of data quality management, involving all stakeholders and coming from a single point of data truth.
The Fourth Industrial Revolution and the Need for Data Certainty
The first industrial revolution mechanized production using steam.
The second created mass production through the power of electricity.
The third used information technology to automate production.
Now a fourth industrial revolution is building on the third.
It is characterized by a fusion of technologies. The velocity, scope and impact of current breakthroughs is exponential. They transform entire systems of production, management, and governance.
To achieve that, they are reliant on data. Gargantuan amounts of data. Data that needs to be 100% accurate, 100% complete, secure, and be augmented at scale to help corporations, industries and governments make smart decisions, fast.
The Importance of Data Quality
Data quality has a tremendous impact on the trajectory an enterprise takes:
- Predicting customer expectations
- Assisting with effective product management
- Being available on-demand to influence top-down decision making
- Tailoring innovations by investigating customer behaviour
- Providing organizations with competitor information
However, there’s one big caveat — if your data isn’t accurate, complete and consistent, it can lead to major missteps when making business decisions. Gartner estimates the average financial impact of poor data quality on businesses at $15 million per year.
Data quality represents a range, or measure, of the health of the data pumping through your organization. For some purposes, and in some businesses, a marketing list with 5 percent duplicate names and 3 percent bad addresses might be acceptable. But if you’re meeting regulatory requirements, the risk of fines demands higher levels of data quality.
The use of manual processes to ingest, clean, transform and migrate data, is prone to error. A call centre operator that misspells a name or postcode, or duplicates orders or invoices, creates an inventory where codes have been incorrectly entered. Multiply this by the billions of bytes of data in the organization, and the risks become huge.
Data quality management provides a context-specific process for improving data quality for analysis and decision making. The goal is to create insights into the health of the data using various processes and technologies on increasingly bigger and more complex data sets, with the goal of creating data truth, based on certainty. This can only be achieved by assuring 100% of the data, through 100% of the journey, 100% of the time.
But is that a realistic goal? With the right methodology, tools, and an understanding of total data quality management, the answer has to be, of course, yes – the alternative results include poor decision-making, cost, lack of control, and increased exposure to risk.
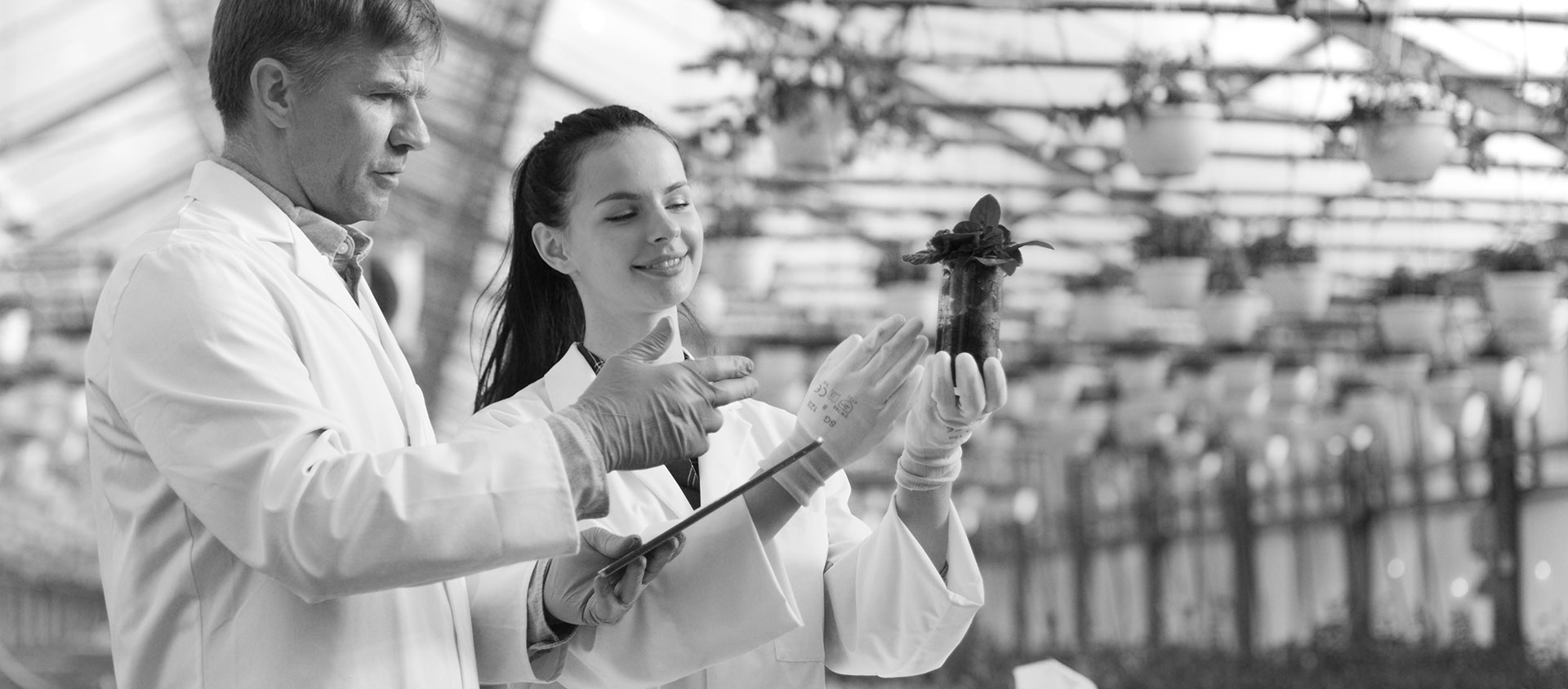
What is Data Quality Management?
Data quality management (DQM) refers to a combination of the right people, methodologies and toolkit, aimed at improving the measures of data quality that matter most to any enterprise organization.
The ultimate purpose is not just to improve data quality, but rather to achieve the outcomes that depend upon high-quality data. Any system – an ERP, CRM, production management or help desk systems is only ever as good as the information it contains.
Data Quality Use Cases
Within any organization, there are seven key use cases for data quality:
1. Enterprise Operation: For example, in relation to sales, finance, supply chain management and HR
2. Data Integration: Connecting systems and data pools to ensure a single point of truth
3. Data Migration: Cloud migration, legacy systems, digital transformation and composable architectures
4. Master Data Management: As it applies to customers, products and employees
5. Data Governance: Regulatory requirements, privacy & business logic
6. Data Analytics: 360 view of customers, products and people
7. AI and Machine Learning: Algorithm and model training, augmented by human intelligence
Data Quality Definition
The most critical points of defining data quality may vary across industries and from organization to organization.
But defining these rules is essential to the successful use of business intelligence software.
Your organization may wish to consider the following characteristics of high-quality data in creating your data quality definitions:
- Integrity: how does the data stack up against pre-established data quality standards?
- Completeness: how much of the data has been acquired?
- Validity: does the data conform to the values of a given data set?
- Uniqueness: how often does a piece of data appear in a set?
- Accuracy: how accurate is the data?
- Consistency: in different data sets does the same data hold the same value?
Effective data quality management requires a structural core that can support data operations. Data quality on its own, however, is considered by many to be too static a definition, a little “old school”.
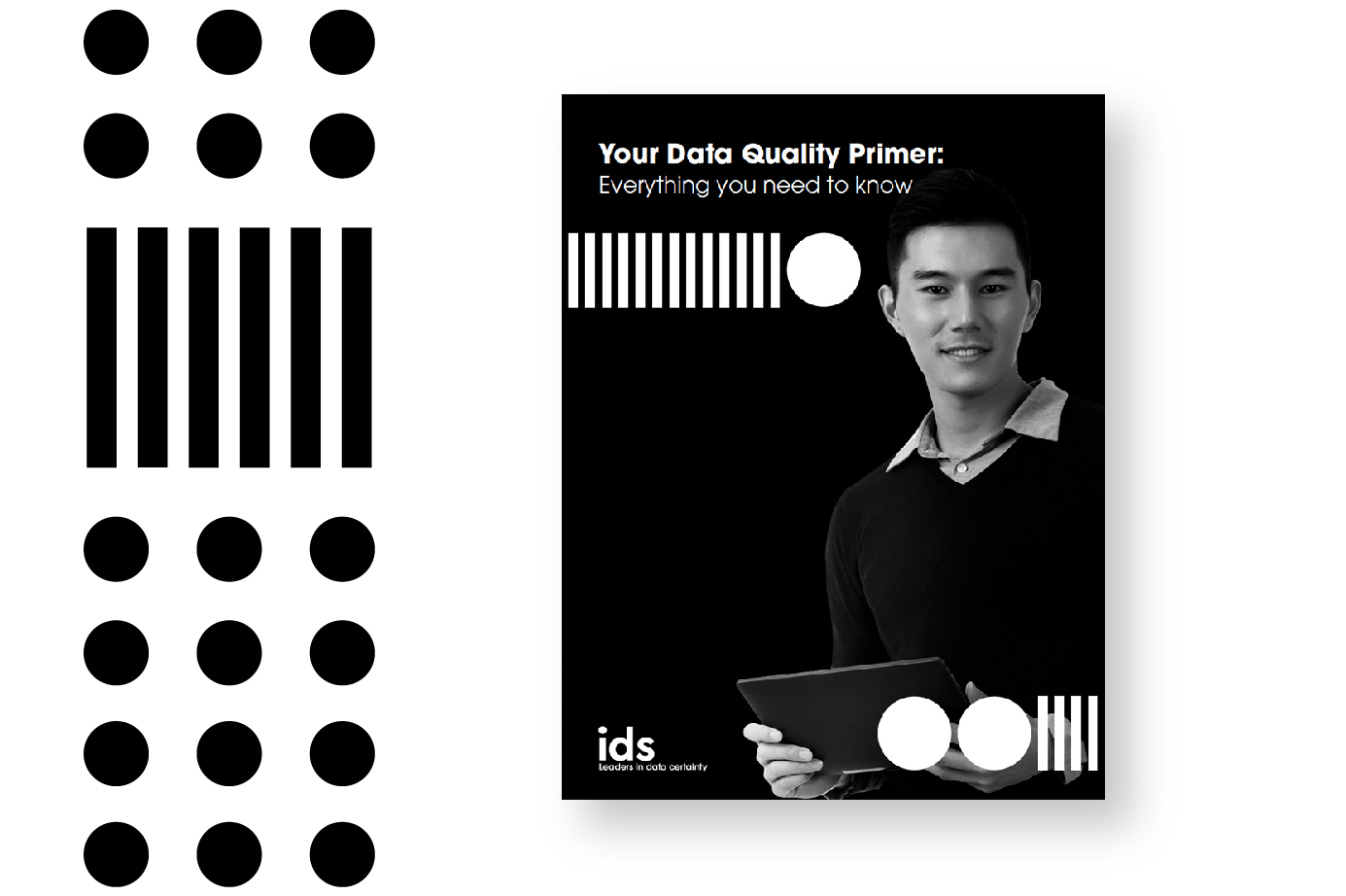
FEATURED CONTENT | DOWNLOADABLE
Everything all businesses - no matter the industry - should understand about data quality
Total Data Quality Management
Modern data quality management (DQM) includes four levels: as organizations become more complex and more sophisticated, both the accessibility of, and the requirement for, all four levels, increases.
Data Quality Management - The Four Levels of Data Quality
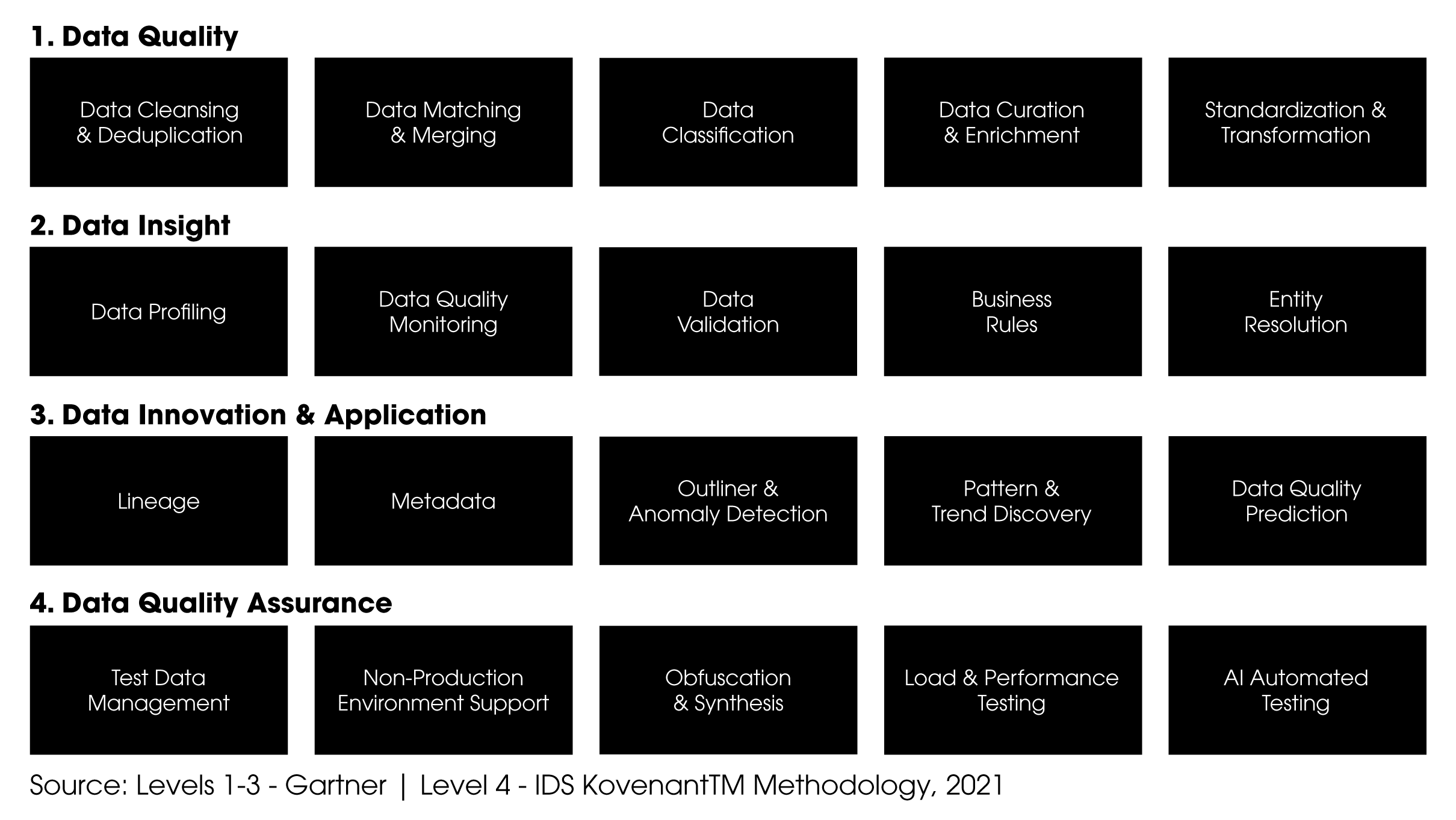
Level #1: Data Quality
This is the structural core of data quality: without this, the organization will struggle to make even the most basic of decisions: there will be mistakes made, and the exposure to risk is high. Core components of data quality include data cleansing and deduplication; data matching and merging: data classification; data curation and enrichment; data standardization & transformation.
To ensure these characteristics are satisfied each time, basic governance principles need to be applied to any DQM strategy:
-
Accountability: Who's responsible for ensuring DQM?
-
Transparency: How is DQM documented and where are these documents available?
-
Protection: What measures are taken to protect data?
-
Compliance: What compliance agencies ensure governance principles are being met?
Once potentially bad or incomplete data has been sorted out by business intelligence tools, such as those within the iData toolkit, the requirement is to complete data corrections such as completing the data, removing duplicates, or addressing other issues.
Level #2: Data Insight
The focus is no longer just on data quality, but on the level of insight that data truth offers, to support strategic decision-making.
This is all about taking meaning from data: turning facts into information and insight. Core elements of the data insight toolkit include data profiling; data quality monitoring; data validation; business rules; entity resolution.
Data profiling audits ensure data quality against metadata and existing measures and standards, to stay ahead of the competition.
Level #3: Data Innovation & Application
Digital transformation is inevitable. However, change can no longer be seen as a one-off event, but a continuum. Data innovation and transformation needs to keep pace. It’s like painting the Forth Bridge – long before you reach the end, you will need to start over. In this context, data innovation and application are required.
Core elements include: establishing lineage, metadata and visualization of metadata; outlier and anomaly detection (potentially through the use of AI), pattern and trend discovery and data quality prediction.
Level #4: Data Quality Assurance
It is no longer enough to assure data in situ, in a static environment. Data quality assurance is the fourth element of the data quality equation, automating testing and ensuring that the data is fit for purpose, not only at a given moment, but as part of a continuously flexing data fabric, or continuum, characterized not just by a large-scale digital transformation, but by micro-shifts in every second of every day.
Core elements include: test data management; data obfuscation; data synthesis; non-production environment support; AI automated testing; load & performance; test delivery management.
Learn more about the iData Toolkit and book a FREE discovery call:
The Data Security Overlay
As data moves, it needs to be kept secure. Constantly shifting data creates a field day for cyber terrorists and others that could benefit from accessing confidential information: medical records, consumer data, political, research, scientific, financial, and people-based information.
In this scenario, data trust is paramount. For this reason, IDS has made quality assurance and testing an integral part of our Kovenant™ methodology. With Kovenant™, we assure 100% of the data, through 100% of the journey, 100% of the time.
We call this data certainty.
Learn about the IDS Kovenant™ Methodology in this video:
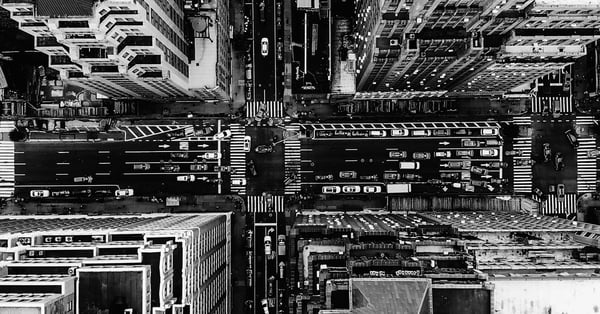
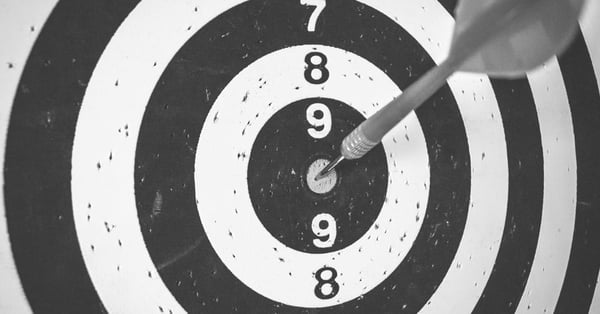
The Real Cost of Bad Data
Citations
How to Create a Business Case with Data Quality Improvement by Susan Moore, Smarter with Gartner, June 19, 2018? https://www.gartner.com/smarterwithgartner/how-to-create-a-business-case-for-data-quality-improvement/