The Impact of Data Quality Problems in Healthcare
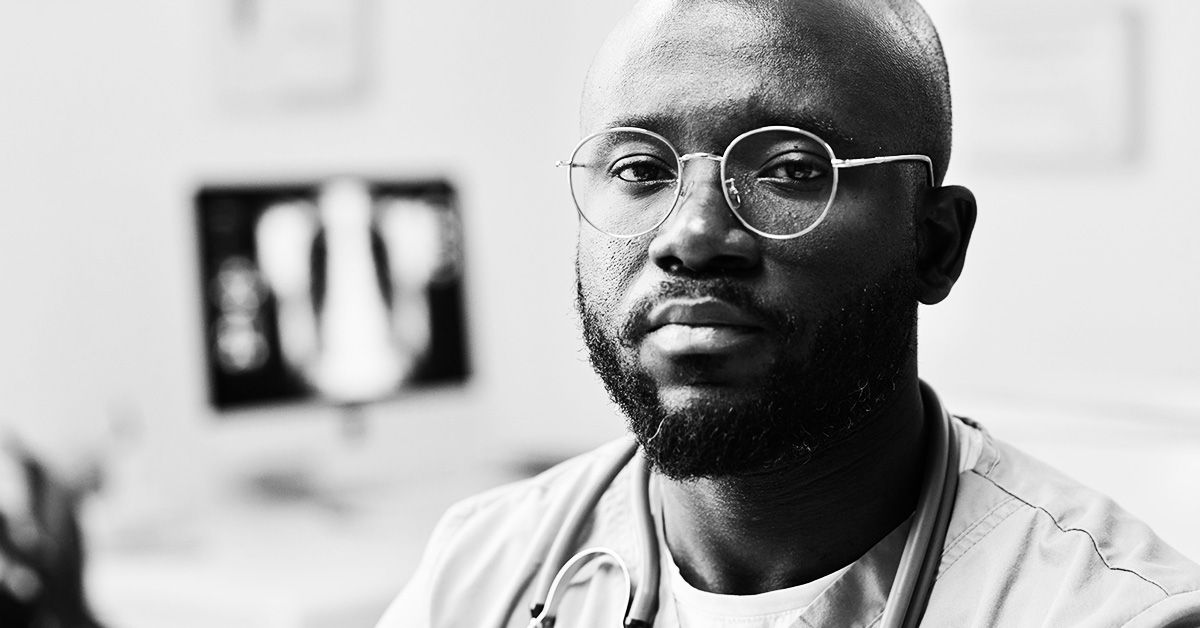
The impact of poor data quality is persistent in healthcare. It affects not only the organization, but also the patient's experience.
Data quality in healthcare is important because it allows trusts and practices to measure their performance and continually improve their policies, practices, and patient care. Strict data quality management can ultimately, take back control of data to mitigate potential risks in the future.
Consequently, the more accurate and up-to-date patient data stored, the better treatment they will receive, and the stronger the organization’s reputation becomes.
Why Data Quality is Essential for Healthcare
When operating with large volumes of sensitive patient information, healthcare organizations must have a comprehensive approach to securing their data and preventing breaches and compliance issues. These include implementing strong encryption techniques and ensuring that all relevant personnel are aware of security protocols.
On the other hand, data quality problems result in inaccurate information about an individual to prescribe them incorrectly.
Yet, further to this, poor healthcare data can be dangerous for patients.
The impact of poor data quality can lead to inaccurate diagnoses, delayed treatments and even death of patients due to improper information. It’s through this chain of data quality problems that accurate records can be the difference between life and death.
The Impact of Poor Data Quality in Healthcare
Patient Frustration and Mistreatment
Poor data integrity is a common problem in healthcare data. When data is entered incorrectly or incompletely, it needs to be fixed or researched manually for the correct records.
This can lead to delays and frustrations among patients needing urgent care. Additionally, data quality issues festering in databases result in mistreatments and prescribing the wrong medication, or none at all. Desperate patients receive poor experiences and suffer life-threatening consequences later.
Employee Frustration and Distrust
As well as impacting patients, the problem with poor data quality in healthcare is that it causes internal stakeholders to become frustrated as employees begin to distrust the data they’re given.
In addition to basic morale issues, this can lead employees to fall back to manual reporting and analysis. Error is then re-introduced and effective, valuable data collection is much harder to achieve.
Employee Inefficiency
38% of healthcare institutions currently have 31 or more disparate systems in their tech stack. It’s no wonder data quality in healthcare organizations is difficult to manage, with disparate systems storing large volumes of healthcare data from multiple digital sources.
This makes solving data quality issues very costly and difficult and time consuming to identify the proper records. It’s nearly impossible to manually ensure that all records are consistent, accurate and reliable across all systems. Healthcare providers, therefore, cannot efficiently deliver value-based care without the right information about their patients' health conditions.
Poor Policy Decisions
As more healthcare policy-makers turn to large quantities of healthcare data to make smarter decisions, it becomes more important that the analytics driving these decisions are accurate.
Due to many business decisions being made off aggregated and transformed datasets, any initial data entry errors lead to problems when managed by other users or systems downstream over a long period of time.
How to Improve Data Quality in Healthcare
Integrated Data Analytics
As mentioned, good data quality in healthcare can support analytics for better policy-making.
When considering how to improve data quality, healthcare organizations should invest in tools that help them enforce data governance practices.
Integrated data analytics help healthcare organizations monitor and maintain data quality to provide better care for their patients.
Using the Correct Tools and Metrics
A potential lack of interoperability between different systems and providers can also be the impact of poor data quality. Bad data can lead to duplications in healthcare records which are challenging to then maintain.
It's very important to make the right choices when it comes to your data analysis tools because they will largely dictate your data analysis strategy.
Without proper thoughts on the tools to use when considering the question in how to improve data quality, you cannot take full advantage of your data.
Using a Suitable Format
One of the most fundamental ways in how to improve data quality for healthcare organizations is constructing a proper format for data management.
Data profiling is necessary to format data to prepare for seamless digital transformation. This helps healthcare businesses understand their data better, identify errors and fix them before data quality issues cause worse consequences later.
Profiling can be done manually, but it’s recommended to seek the help of automated tools. It’s important to use a tool that will not only profile data, but also map and cleanse it to be used in a comprehensive format and utilized seamlessly across different platforms.
A HBR assessment discovered only a 55% data quality score attributed to healthcare data. This is worryingly low for an industry operating with such sensitive personal information and offering highly essential services.
IDS do not settle for less than 100% data quality.
The Kovenant™ methodology has been pioneered by IDS’ data certainty team to assure 100% data quality, 100% of the time.
Find out how this data certainty and assurance framework can rekindle your healthcare business’ data, solving data quality problems and sustaining a high-quality score.

100% Data Assurance for a Healthcare Organization
How iData performed rapid implementation of data obfuscation to eliminate risk and reduce the cost of delivering test data at scale.
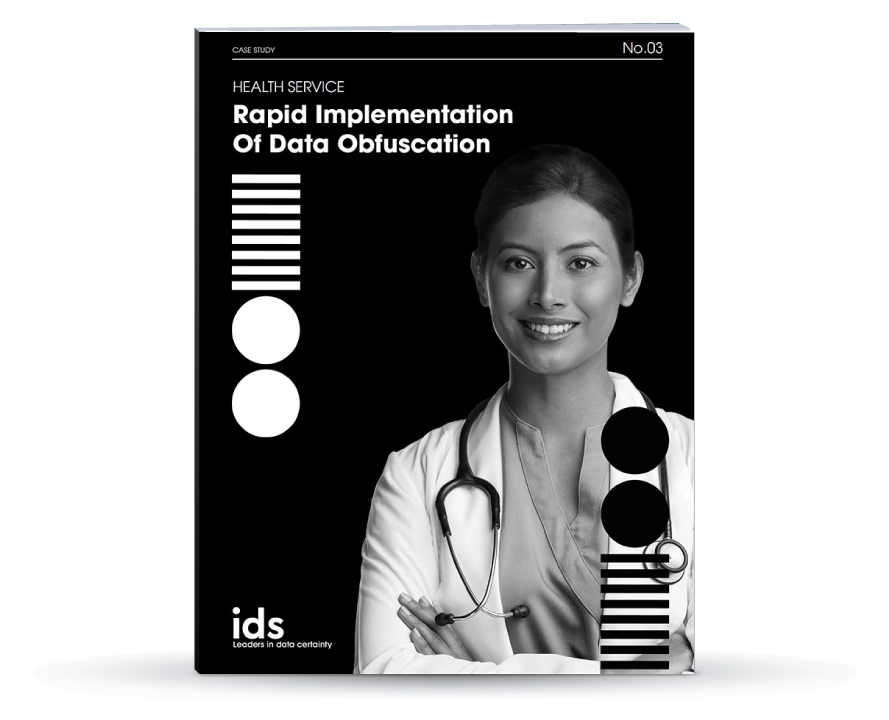