Why Data Quality is so Important to Your Big Data Architecture
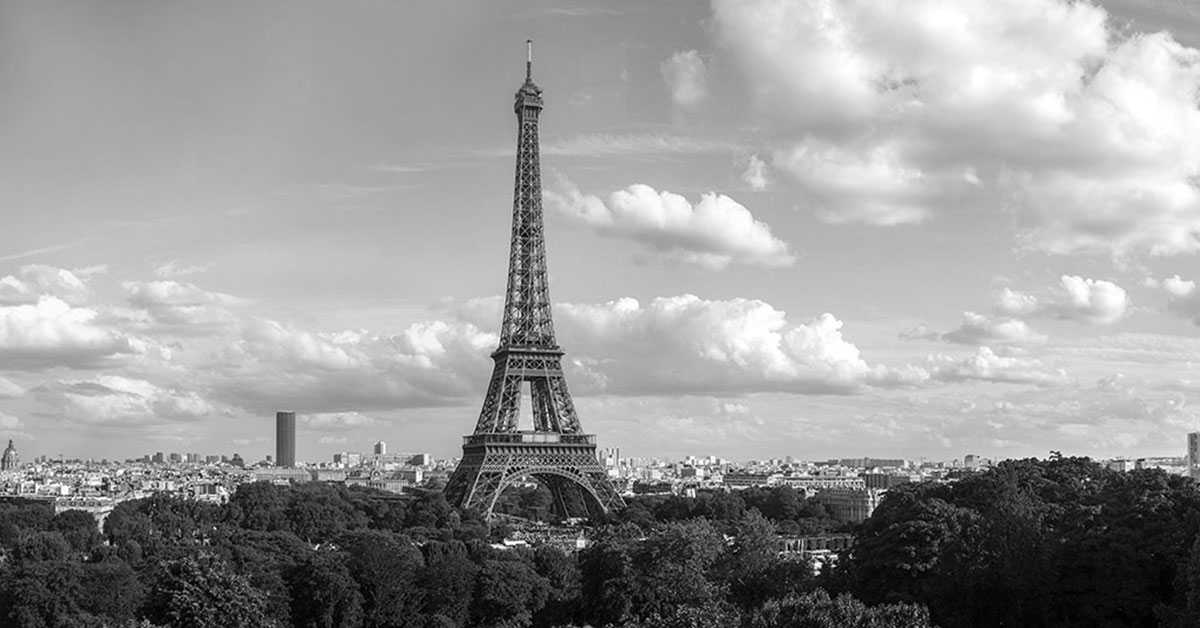
Why Data Quality is so important to your big data architecture solution.
Big data is crucial for any business and the analysis of it often enables businesses to make informed business decisions. A well thought out big data architecture can save your company money and help predict future trends to enable the most concrete business decisions.
A good place to start is what is big data architecture? It is the predominant system used to process vast volumes of data from multiple sources, which is often referred to as ‘big data’ due to its sheer size.
The architecture can be considered the blueprint for a big data solution based on the business needs of an organization, and is designed to handle the following types of work:
• Batch processing of big data sources
• Real-time processing of big data
• Predictive analytics and machine learning
The benefits of big data architecture
The volume of data that is available for analysis grows daily, with more sources than ever for this data collection. But having all this data is only half of the equation, the data also needs to be of the highest quality for your business to get the most of your analysis. You also need to be able to make sense of the data and can use it in time to impact critical business decisions.
Ultimately using a big data architecture can have many benefits to you, including:
• Reduce costs – Big data technologies such as Hadoop and cloud-based analytics can significantly reduce costs when it comes to storing large amounts of data.
• Make faster, better decisions – using the streaming component of big data architecture, you can make decisions in real time.
• Predicting future needs and creating new products. Big data can help you to gauge customer needs and predict future trends using analytics.
Of course, as suggested above, the quality of the data you have will have a material impact on all the perceived benefits of a big data architecture. Poor data quality will drastically hamper good intentions and benefits, even if your big data architecture solution is right!
This leads very nicely onto the challenges of big data architecture. When all the key elements are right a well-conceived and executed big data architecture can save your company money and help predict important trends, but it is not without its challenges. Be aware of the following issues when working with big data.
1. Data Quality
Anytime you are working with diverse data sources, data quality is always going to be a challenge. This means that you’ll need to do work to ensure that the data formats match and that you don’t have duplicate data or are missing data that would make your analysis unreliable. You’ll also need to analyse and prepare your data before you can bring it together with other data for analysis. iData can help you achieve data quality.
2. Scaling
The value of big data is in its volume however this can also become a significant issue. If you have not designed your architecture to scale up, you can quickly run into problems. Your costs of supporting the infrastructure can mount if you don’t plan for them. This can be a burden on your budget. Equally, if you don’t plan for scaling, your performance can degrade significantly. The good news is, both issues can and should be addressed in the planning phases of building your big data architecture.
3. Security
A concern when there are vast amounts of data, and while big data can give you great insights, it’s challenging to protect that data. Fraudsters and hackers can be very interested in your data, and they may try to either add their own fake data or skim your data for sensitive information. Key here is to secure the perimeters, encrypt your data, and work to anonymize the data to remove sensitive information.
Is there such a thing as a typical big data architecture?
Not really, as a big data architecture varies based on a company’s infrastructure and needs, however there are core components that tend to have commonality on a case by case basis which include:
Data sources. All big data architecture starts with your sources. This can include data from databases, data from real-time sources and and static files generated from applications.
Data store. You’ll need robust storage for the data that will be processed via big data architecture. Often, data will be stored in a data lake, which is a large unstructured database that scales easily.
You will need to handle both real-time data and static data, so a combination of batch and real-time processing should be built into your big data architecture. This is because the large volume of data processed can be handled efficiently using batch processing, while real-time data needs to be processed immediately to bring value.
Batch processing involves long-running jobs to filter and prepare the data for analysis.
Analytical data store – after you prepare the data for analysis, you need to bring it together in one place so you can perform analysis on the entire data set. The importance of the analytical data store is that all your data is in one place so your analysis can be comprehensive, and it is optimized for analysis rather than transactions. This might take the form of a cloud-based data warehouse or a relational database, depending on your needs.
Analysis or reporting tools – once ingesting and processing various data sources, a tool to analyse the data is required. Frequently, BI (Business Intelligence) tools will be used here and it may require a data scientist or data scientist resources to explore the data further.
Automation – moving the data through these various systems requires orchestration usually in some form of automation. Ingesting and transforming the data, moving it in batches and stream processes, loading it to an analytical data store, and finally deriving insights must be in a repeatable workflow so that you can continually gain insights from your big data. Repeatability is key here, without expending time and money to do so.
As we mentioned earlier, in every big data architecture solution and planning, data quality is a critical consideration.
You will need to clean your data and securely get it into one place. Bearing in mind, obviously, that big data means potentially enormous volumes of data.
iData can help. iData is an automated solution that can handle vast volumes of your data at speed and deliver total coverage of all your data. iData cleanses, validates, securely moves and monitors your data at speed. So, you gain repeatable, data quality governance process and total data quality coverage.
Contact us today to find out more!
Ready to discover iData?

Performing Automated Testing to Halve Migration & Transformation Time
Partnered with an award-winning ERP migration consultancy, IDS accelerated the time to migrate and transform a healthcare company's data by using the iData toolkit.
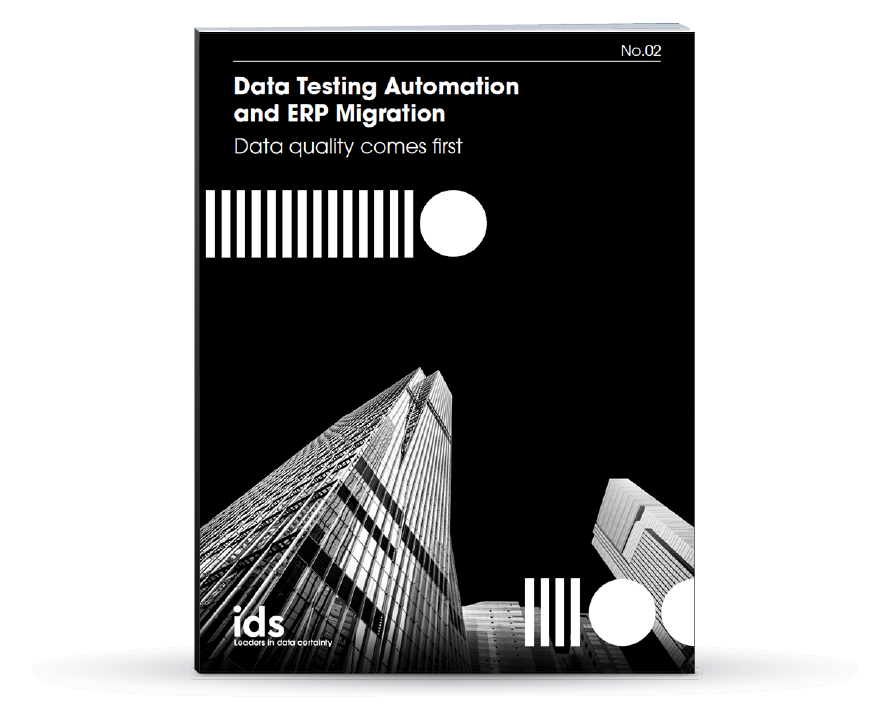