Best Practices to Improve Data Quality for Decision-Making
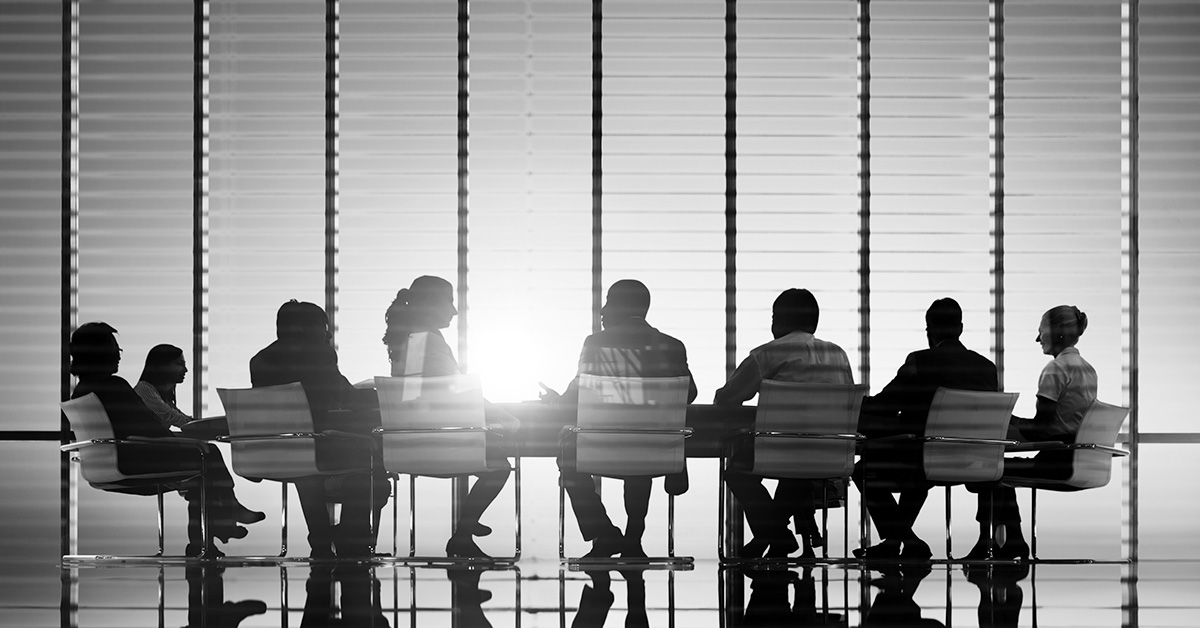
Challenges to Improve Data Quality for Strategic Decision-Making
Business leaders need to take pragmatic and practical steps to ensure that data quality management standards are embedded at the heart of their operations.
The challenge for businesses is not knowing the exact quality of their data before embarking on a digital transformation. Working with duplicated, incomplete and inaccurate information is a fatal risk. Organizations must be proactive with their data quality management strategy to avoid any potential issues throughout their transformation.
The first challenge is that businesses often start a digital transformation with ambiguity around the quality of their data. This obvious risk is increased when project teams are unaware of the lack of data quality, for example, if they have any missing values or if they have errors in their data set.
Further, project teams may not know if their data set has any biases or if it's skewed with one perspective on an issue. Therefore, data quality must come first in any digital transformation or migration.
It’s essential to conduct analysis to identify missing or similar records. It is fundamental to rely on data that is accurate to have any chance of success.
The second challenge is that data quality analysis takes time, causing time lags in measuring KPIs and making decisions, ultimately affecting the bottom line. This problem is compounded by the fact that data quality analysis often relies on manual processes.
With poor quality data now estimated to be costing businesses around $12.9 million annually it means that no enterprising organization can afford to wait for these manual processes before implementing strategic decisions.
Introduce a data quality mindset into operational structures. Follow a step-by-step, structured approach, and a mature, data quality-centric governance program will deliver ‘better leads, better customer understanding and better customer relationships’ to achieve a sustainable competitive advantage.
4 Key Steps to Improve Data Quality for Decision-Making
1 - Laying the Foundations
The first practical suggestion involves identifying the clear connections between your business processes, key performance indicators (KPIs), and data assets.
Data quality is a concern for many businesses. It's not just about accuracy and completeness; it also involves timeliness, consistency, and validity. It can be difficult to track and measure the quality of data, and it's also challenging to assess what the first steps are to improve it.
Data assets are the foundation for any company’s data quality efforts. Data assets must relate to business processes to compound improvements from one business area to another.
These assets can be anything from spreadsheets to databases, to files on hard drives or servers. Once these connections are made, KPIs should be mapped against them to identify which processes need improvement, and which ones are working well.
Make a list of all the existing data quality issues your organization is facing and how they are impacting revenue and other business KPIs.
2 - Defining a Fit-for-Purpose Action Plan
Every business is different, and it's important at the outset to define what is a “best fit” for your organization. It is necessary to create an action plan with relevant stakeholders on how they will go about analyzing the data and identifying any issues within it.
It is also important to remind project teams of the established KPI's, to demonstrate that their efforts are paying off.
Quality assurance must be part of any action plan. Compare the data with past reports or with other sources of information to ensure it is analysis-ready and error-free.
Equally, data profiling, should be arranged as an ongoing process by examining and summarizing data from existing sources. This is critical in helping you identify and prioritize which data quality issues are to be fixed and when.
A typical example might be identifying missing contact information in customer records that, if not addressed urgently, could result in poor customer relations, harming your business’ future.
3- Assigning Data Quality Accountability
The importance of data quality has been neglected for a long time. It's time to change that and assign responsibilities, as well as accountability, to ensure enterprise-wide compliance and success.
At each board or executive and senior management team meeting, data quality should be an agenda item. There is a need for data governance and compliance to ensure that the company is also compliant with local and industry regulations. Data governance and compliance are two different things, but they are closely related.
The Board of Directors can assign these responsibilities, to a Chief Data Officer who will ensure that there is a robust policy in place to maintain data quality.
When all departments comply with the company’s data standards, it improves the accuracy of information across the entire organization, lessens risk, and provides greater visibility into operational systems, networks, applications, and databases.
4 - Embedding Data Quality in the Organizational Culture
As the final stage of Gartner’s route to data quality maturity, data quality must be embedded into an organization at a cultural level.
Data quality is not a one-time event, it's an ongoing process. To drive successful outcomes it must be embedded into organizational cultures.
Dashboard meetings are a great way to monitor the performance of data quality. They should be held on a regular basis and should cover all aspects of data quality to communicate tangible business improvements across departments.
Constructive dashboard sessions can help support senior stakeholders with more informed, profitable decisions to help the business run smoothly and maximize chances of future success.
As well as internal adaptations, connecting with external peer groups is also encouraged to foster an exchange of alternative perspectives on best practices and insights into the approaches that others are taking to address similar challenges.
Helping Organizations Sustain Data Quality Standards
Our flagship software and data quality services are used by government, institutes and multinational companies, to resolve data quality issues and improve data quality initiatives.
IDS are leaders in data certainty and are so-called for making sure our clients’ data is accurate and up-to-date and equipped with a robust strategy, 100% of the journey, 100% of the time.
We will work with your team and provide consultative advice in the field of data governance and digital transformation to make sure the right people are overseeing the right projects. Implementation partners and consultants will provide insights on how to improve the quality of your data and detect anomalies in the system and much more.
If you want to know more about how IDS can support your company with its data, contact us for a free consultation today.

Your Data Quality Management Health Check
Learn more about the techniques IDS uses to help with your data quality assurance including data cleaning, standardization and profiling so your data is ready for change.
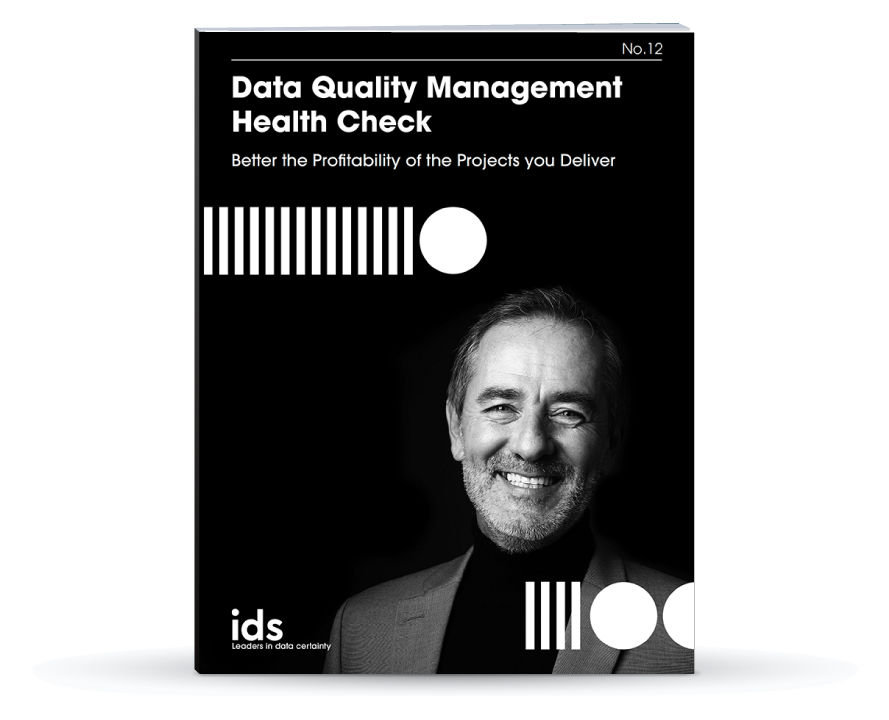