How to Get Started With Data Quality - For Everyone
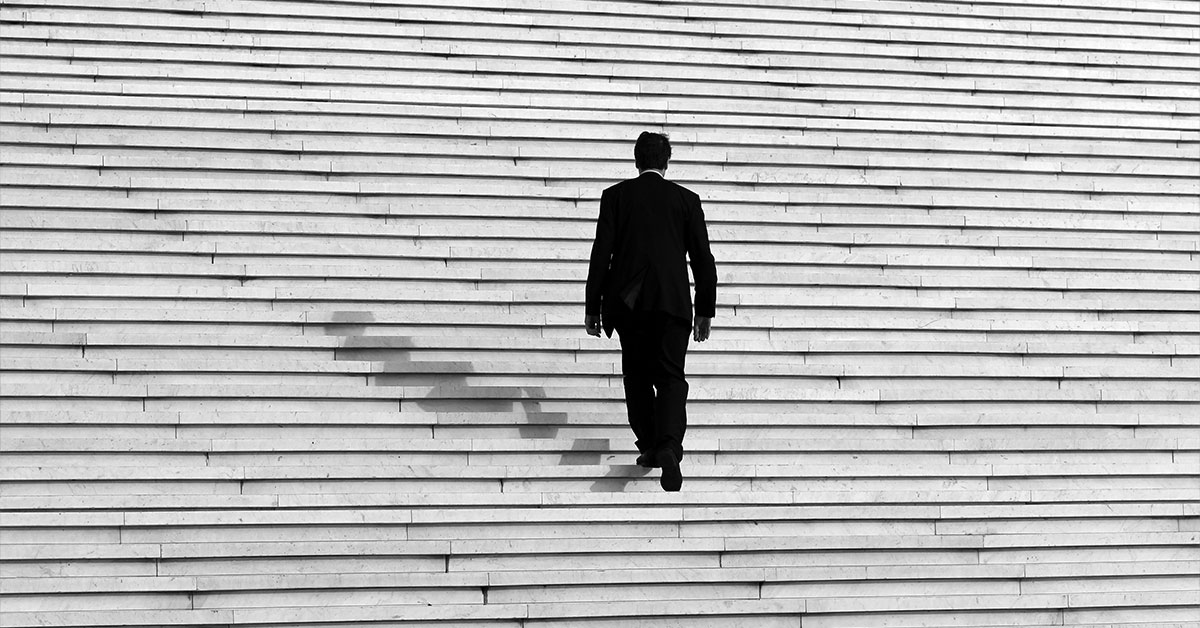
Companies rely on data to make strategic decisions, support consumers, create schedules, and handle other important activities. Data-driven activities are not a transient business practice but more of a new phenomenon. The company’s choices could result in menace if it’s not based on full and reliable data. After all, data is the backbone of activities, driving financial and non-financial important business decisions. The quality of data is not something that can be changed fundamentally by identifying and solving issues. Instead, organizations can start by generating data of high quality.
The purpose of this article is to give you a better understanding of data insights on how to build data from the beginning that produces and maintains good data quality.
Firstly, we must understand what data quality means. Data is of excellent quality when it meets the criteria of its intended purpose for customers, decision-makers, and downstream frameworks. Data quality teaches a realistic approach to the development, enhancement, and management of information quality that is essential for the distribution of goods and services, customer loyalty, and targets for any organization. This data quality concept is wide enough to allow organizations with diverse products, markets, and objectives to consider if their data meets the requirements. Additional dimensions of data that can be used by organizations to evaluate if their data matches their targeted use include:
Consistency:
The data categories must be consistent with the expected iterations of the data being obtained.
Accuracy:
Data obtained must be precise, relevant, and correctly portrays what it should be.
Relevance:
Not only should data be accurate, but it also has to be relevant to a company’s needs and purposes. Companies waste precious database storage if they obtain information that is foreign to their function, and irrelevant data will also impede identifying key customer targets in monitoring and analyzing.
Completeness:
This helps ensure that there were no data gaps between what was to be obtained and what was eventually gathered.
Validity:
Validity is generated from the process rather than the outcomes.
Based on the specification and the existence of the data itself, the criterion for good data quality varies. For example, a company’s core consumer dataset has to follow quite strict expectations for the requirements mentioned earlier. In contrast, a third-party data source may have more tolerance for inconsistencies or incompleteness. In order for a company to produce high-quality data, each data storage generated in the process from the beginning to the end must be handled and monitored.
If an organization can handle each dataset’s data quality at the time it’s generated, the data quality is automatically assured. Here are some points you must adhere to manage good data quality:
- Comprehensive profiling of data and regulation over incoming data.
- Accurate collection of data specifications.
- Careful layout of the data system to prevent duplicated data.
- Automated checking of regression as part of change management.
- Regulation of data confidentiality.
If you need help, iData is here! You can also check out the Getting Started with Data Quality and Advancing Your Career in Data courses from the iData Quality Academy.

The Ultimate Beginner's Guide to Data Quality
The Data Quality Primer from the iData Academy will enable anyone from any profession on the importance of data quality and its impact on YOUR industry.
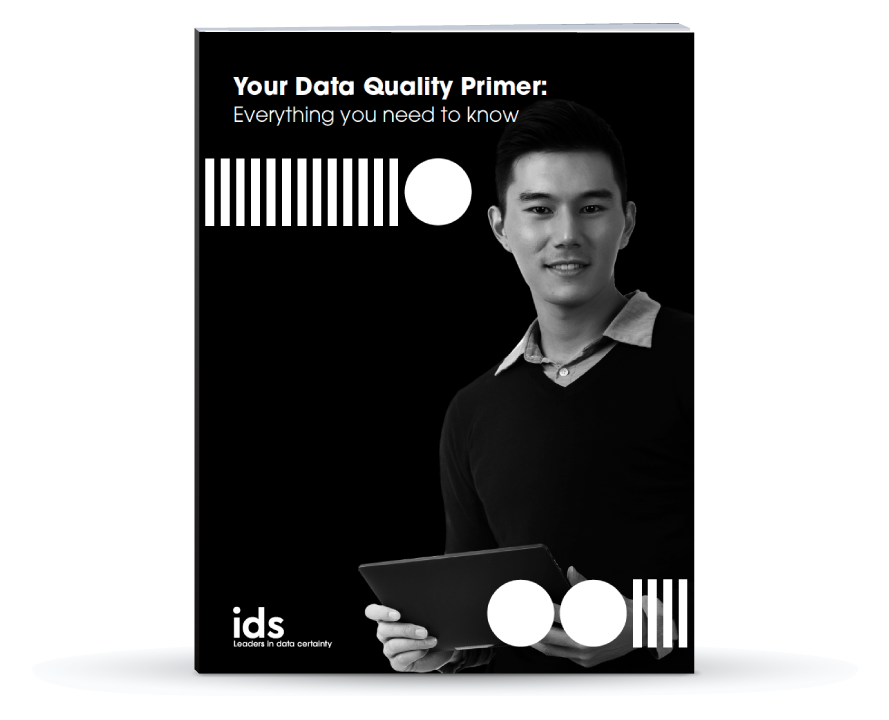